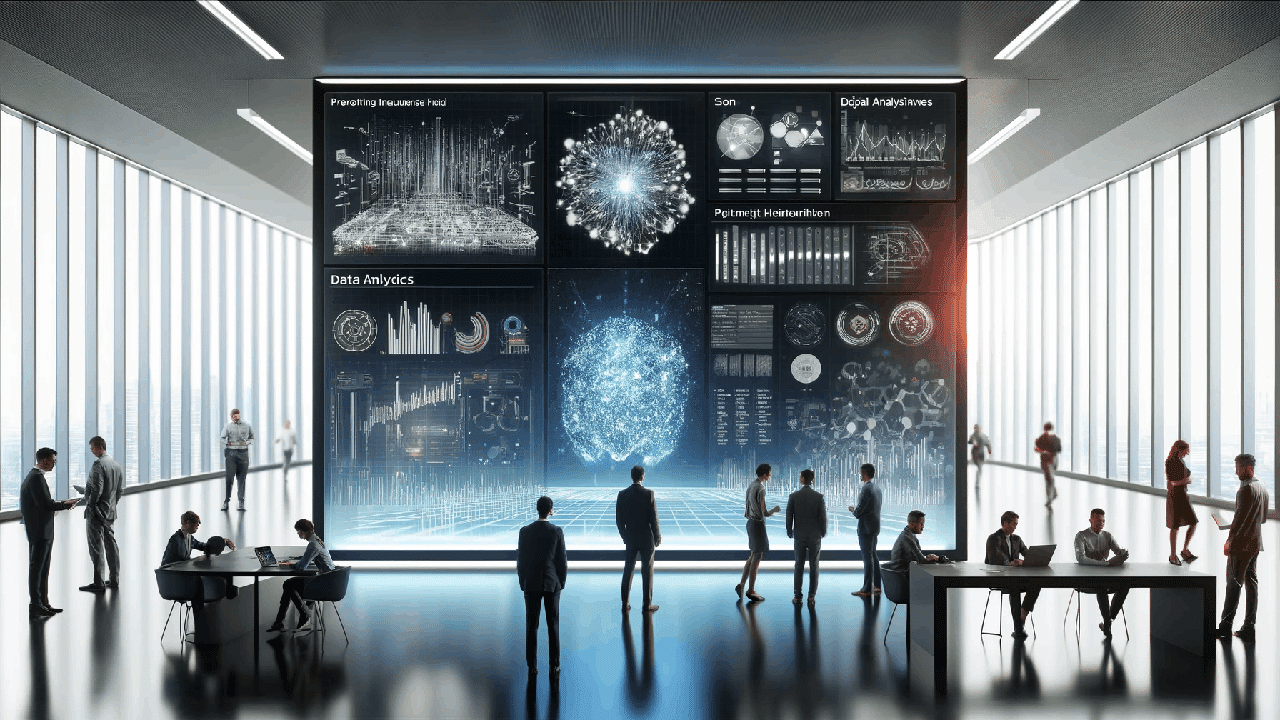
Learn how deep learning technology is revolutionizing the fight against insurance fraud.
Preventing Insurance Fraud with Deep Learning
Insurance fraud costs the US about $308.6 billion annually. After tax evasion, it is the second most costly white-collar crime in the US. This is a significant issue that affects both the insurance industry and consumers. Fraudulent claims cost insurance companies billions of dollars yearly, increasing premiums. For example, an average insurance holder loses up to $900 due to fraud-induced premium rates.
No wonder insurance companies invest actively in AI-based security software and solutions. For example, Lemonade insurance provider, which operates in the United States and the UK, has deployed chatbots to handle insurance claims. It let the company double its revenue (from $67 million in 2019 to $128 in 2021) in a couple of years.
AI-based solutions also have great potential in battling insurance fraud. In this article, we shall discuss what insurance fraud is and how it can be solved with the help of deep learning and AI.
Let’s get started!
Understanding Insurance Fraud
Before delving into the role of deep learning in fraud detection, it is essential to understand the scope and common types of insurance fraud.
Whether it’s staged car accidents, property damage, or submitting wrongful information during filing a medical insurance form, insurance fraud encompasses any act committed to obtain undeserved benefits from an insurance policy.
According to statistics, in the US, the most common type of insurance fraud is medical fraud, which is estimated at $105 billion annually. It is followed by life and property insurance fraud.
Insurance fraud is a complex and multifaceted issue that poses significant challenges to insurance companies and law enforcement agencies alike.
Insurance fraud perpetrators can range from individual policyholders looking to make a quick profit to sophisticated criminal organizations orchestrating large-scale fraud schemes. Detecting and preventing insurance fraud requires a combination of advanced technology, data analytics, and investigative techniques.
Interested to check how AI can help detect fraudulent activity?
Contact eNest for a free consultation and advice. Our team will provide you with all the necessary information on building your custom AI solution, how much it will cost and how long its development will take. Book a call to learn more now!
The Scope of Insurance Fraud
Insurance fraud is a pervasive problem that affects various insurance sectors, including health, auto, and property insurance. According to industry estimates, insurance fraud accounts for approximately 10% of all claims made, which translates to billions of dollars in losses each year. The impact of insurance fraud extends beyond financial losses, leading to higher premiums for policyholders, increased operational costs for insurers, and a general erosion of trust in the insurance industry.
Common Types of Insurance Fraud
There are several common types of insurance fraud that insurance companies and investigators must remain vigilant against:
- Staged accidents: Fraudsters deliberately cause accidents or stage elaborate scenarios to file fraudulent claims.
- Exaggerated injuries: Individuals may exaggerate or fabricate injuries to receive higher compensation.
- False documentation: Fraudsters submit falsified documents, such as medical records or repair invoices, to support their fraudulent claims.
Additionally, other forms of insurance fraud include premium diversion, where insurance agents embezzle premiums instead of remitting them to the insurer, and ghost policies, which involve selling fake insurance policies to unsuspecting customers. By understanding the various tactics used by fraudsters and staying informed about emerging fraud trends, insurance companies can better protect themselves and their policyholders from falling victim to fraudulent activities.
The Role of Deep Learning in Fraud Detection
Deep learning is a subset of artificial intelligence (AI) that mimics the human brain’s neural networks to analyze and process complex data. In the context of insurance fraud detection, deep learning algorithms can identify patterns and anomalies in large volumes of data to uncover fraudulent activities. To leverage the power of deep learning effectively in this domain, insurance companies may need to hire a data scientist with expertise in machine learning and neural networks.
One of the key advantages of deep learning in fraud detection is its ability to adapt and improve over time. As the system processes more data and encounters new types of fraud, it can continuously learn and enhance its detection capabilities. This adaptability is crucial in combating the evolving tactics of fraudsters who are constantly devising new ways to deceive insurance companies.
What is Deep Learning?
Deep learning algorithms are designed to learn and make predictions or decisions based on large datasets. They utilize multiple layers of interconnected nodes called artificial neurons, which enable the system to extract meaningful features from the input data.
Moreover, deep learning models can automatically identify complex patterns and relationships within the data that may not be apparent to human analysts. This capability is particularly valuable in fraud detection, where fraudulent activities are often disguised and hidden among legitimate transactions.
How Deep Learning Works in Fraud Detection
In the context of insurance fraud detection, deep learning algorithms analyze various data sources, including claim forms, policyholder information, and historical claims data. By considering multiple data points simultaneously, deep learning models can identify fraudulent patterns that may be difficult for traditional rule-based systems to detect.
Furthermore, deep learning algorithms can detect anomalies in real-time, allowing insurance companies to respond swiftly to potential fraud attempts. By continuously monitoring transactions and activities, these systems can flag suspicious behavior as soon as it occurs, minimizing the financial losses associated with fraudulent claims. To implement such advanced fraud detection systems, insurance companies may hire a software development team with deep learning and data analytics expertise to build and deploy robust fraud detection solutions.
Benefits of Using Deep Learning in Insurance Fraud Prevention
Implementing deep learning solutions for insurance fraud prevention offers several advantages over traditional methods.
Deep learning algorithms provide highly accurate results in identifying fraudulent activities. By leveraging advanced techniques such as image recognition and natural language processing, these models can extract valuable insights from unstructured data sources, leading to more effective fraud detection.
Furthermore, the use of deep learning in insurance fraud prevention not only enhances accuracy but also improves efficiency. These algorithms can process vast amounts of data at a rapid pace, enabling insurance companies to detect and respond to potential fraud in real-time. This real-time monitoring capability is crucial in preventing fraudulent activities before they escalate, ultimately safeguarding the financial interests of both the insurance providers and policyholders.
Cost Savings for Insurance Companies
By incorporating deep learning into their fraud prevention strategies, insurance companies can significantly reduce financial losses caused by fraudulent claims. The ability to detect fraud early and accurately minimizes unnecessary claim payouts, ultimately lowering insurance premiums for policyholders.
Moreover, the implementation of deep learning technologies can streamline the claims investigation process, leading to faster resolution times and reduced operational costs for insurance companies. By automating the detection of suspicious patterns and anomalies in claims data, insurers can allocate their resources more efficiently, focusing on legitimate claims and improving overall customer satisfaction.
Implementing Deep Learning Solutions for Fraud Prevention
Implementing deep learning solutions for insurance fraud prevention requires careful planning and execution. Several necessary steps must be followed to ensure successful implementation.
When embarking on the journey of implementing deep learning solutions for fraud prevention in the insurance industry, it is crucial to consider the diverse nature of fraudulent activities that can occur. From false claims to identity theft, the landscape of insurance fraud is multifaceted and ever-evolving. Therefore, a comprehensive understanding of the different types of fraud schemes is essential in designing effective deep learning models to combat them.
Necessary Steps for Implementation
Firstly, insurance companies need to gather and clean their data, ensuring its quality and reliability. Next, they must select or develop appropriate deep learning models tailored to their specific fraud detection requirements. Companies should also consider integrating their existing systems and processes with the deep learning solution for seamless operation.
Moreover, it is imperative for insurance companies to establish robust monitoring mechanisms post-implementation to continuously evaluate the performance of the deep learning solution. Regular performance assessments and fine-tuning of the models based on real-time data can enhance the accuracy and efficiency of fraud detection algorithms.
Challenges and Solutions in Implementation
While implementing deep learning solutions for insurance fraud prevention can yield significant benefits, it comes with its challenges. One common challenge is the need for large amounts of labeled training data. To overcome this, insurance companies can collaborate with industry organizations to share anonymized data, ensuring compliance with privacy regulations.
Additionally, another challenge in implementing deep learning solutions for fraud prevention is the interpretability of the models. As deep learning algorithms are often considered black boxes due to their complex structures, efforts should be made to enhance the transparency of the decision-making process. Techniques such as model explainability tools and feature importance analysis can provide insights into how the deep learning models arrive at their fraud detection decisions.
The Future of Deep Learning in Insurance Fraud Prevention
As technology continues to evolve, the future of deep learning in insurance fraud prevention looks promising.
Deep learning, a subset of artificial intelligence (AI), has been making significant strides in enhancing fraud detection capabilities within the insurance industry. By utilizing complex algorithms and neural networks inspired by the human brain, deep learning systems can analyze vast amounts of data to identify patterns and anomalies that may indicate fraudulent activities.
Emerging Trends in Deep Learning
New advancements in deep learning, such as generative adversarial networks (GANs) and reinforcement learning, hold great potential for improving fraud detection accuracy and speed. GANs can generate synthetic data that helps train more robust fraud detection models, while reinforcement learning enables systems to optimize their fraud detection strategies over time.
Moreover, the application of natural language processing (NLP) in deep learning models allows insurance companies to analyze unstructured data, such as text from claim descriptions or customer communications, to uncover subtle indicators of fraud that may have previously gone undetected.
Potential Impact on the Insurance Industry
The widespread adoption of deep learning in insurance fraud prevention will likely transform the insurance industry. As insurers leverage advanced analytics and AI technologies, they can proactively identify and prevent fraudulent activities, resulting in improved customer satisfaction, cost savings, and a fairer insurance market.
Furthermore, the integration of deep learning systems with existing fraud detection processes can enhance the overall efficiency of fraud investigations. By automating the analysis of large datasets and flagging suspicious claims in real-time, insurers can expedite the identification of potential fraud schemes and take timely action to mitigate risks.
Summing up
In conclusion, deep learning offers immense potential in the fight against insurance fraud. By harnessing the power of artificial neural networks, insurance companies can better protect their resources and policyholders from fraudulent activities. As technology continues to advance, the implementation of deep learning solutions for insurance fraud prevention will undoubtedly become more prevalent, leading to a more secure and trustworthy insurance industry for everyone.
Want to build a proprietary insurtech solution for your company?
We are here to help! Call eNest for a one-on-one consultation on your business case and calculations on your future project cost and timeline. Book a call now!
Have a question?
Speak to Data Scientist
Jagdeep ChawlaMS in Data Science
NorthWestern Univeristy, Illinois
MS in Data Science
NorthWestern Univeristy, Illinois
More Articles
-
Speech to text solution for document handling in aviation logistics
Discover how the latest speech to text solution is revolutionizing document handling in aviation logistics. Speech to text solution for document handling in aviation logistics Su... more -
Insurance Fraud Prevention via Deep Learning
Learn how deep learning technology is revolutionizing the fight against insurance fraud. Preventing Insurance Fraud with Deep Learning Insurance fraud costs the US about $308.6 b... more -
Driving Efficiency: Computer Vision for Autonomous Vehicles
Explore the potential of computer vision in revolutionizing autonomous vehicles and driving efficiency. Driving Efficiency: Computer Vision for Autonomous Vehicles It’s not jus... more
We are ranked in Top 1%
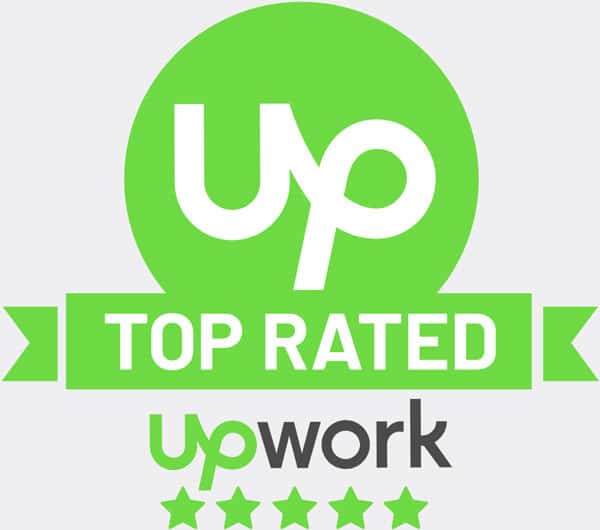
.NET software engineer staffing
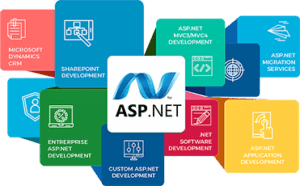
Ready to
Get Started?
Follow Us
Expert Solutions
Privacy Overview
Necessary cookies are absolutely essential for the website to function properly. This category only includes cookies that ensures basic functionalities and security features of the website. These cookies do not store any personal information.
Any cookies that may not be particularly necessary for the website to function and is used specifically to collect user personal data via analytics, ads, other embedded contents are termed as non-necessary cookies. It is mandatory to procure user consent prior to running these cookies on your website.